For the first time in history, a computer has beaten the human world champion at Go.
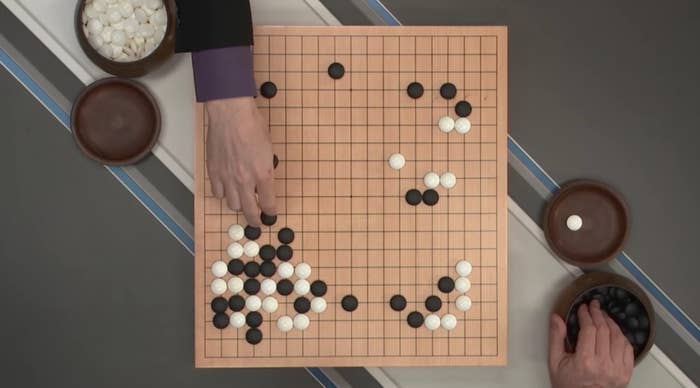
Here's why that's a big deal. First, Go is incredibly complicated – millions upon millions of times more complex than chess.
View this video on YouTube
"It's sheer mathematics," Professor Murray Shanahan, an AI researcher at Imperial College London, told BuzzFeed News. "The number of possible board configurations in chess, of course, is huge. But with Go, it's enormously larger."
In chess, there are on average about 35 to 38 moves you can make at any point. That's called the "branching factor". In Go, the branching factor is about 250. In two moves, there would be 250 times 250 possible moves, or 62,500. Three moves would be 250 times 250 times 250, or 15,625,000. Games of Go often last for hundreds of moves.
It's sometimes said that in chess there are more possible games than there are atoms in the observable universe. In Go, by one estimate, there are something like a trillion trillion trillion trillion trillion trillion trillion more than that. To write the total number out, you'd need to put a 1 followed by 170 zeroes. That's why, nearly 20 years after computers became better at chess than humans, they've only just caught up at Go.
That means that a computer can't just look at every single possible move and pick the best one.
That's called "brute force" processing. "You simply can't use brute force for Go," says Shanahan. "You can't with chess either, but you can tackle it that way a bit, use brute force to search ahead through many, many possibilities. But with Go the number of possible board combinations is enormously larger."
The branching factor means that even a few turns ahead, the number of possibilities becomes too huge for even the fastest computer to search through.
That means that AlphaGo's victory isn't simply a product of computers getting faster and more powerful. Computers will never be powerful enough to brute-force Go. Software is always more important than hardware.
"The general rule of thumb in these areas is that hardware counts for an enormous amount, but software counts for more," Eliezer Yudkowsky, an AI researcher and co-founder of the Machine Intelligence Research Institute (MIRI) in California, tells BuzzFeed News. "If you have a choice between using software from 2016 and hardware from 1996, or vice versa, and you want to play computer chess or Go, choose the software every time."
And that means that AlphaGo has had to use learning techniques that are more like human intuition.
Human players don't follow every possible branch that the game could go. They look at the board and see patterns. "The way that human players play chess or Go or any game like that," says Shanahan, "is that we get to recognise what a good board pattern looks like. There's an intuitive feel for what's a good strong position versus what's a weak one.
"Human players build that up through experience. What DeepMind have managed to do is capture that process using so-called deep learning, so it can learn what constitutes a good board configuration."
Its creators fed it hundreds of thousands of top-level Go games, and then, after it had learned from them, let it play against itself, millions of times.
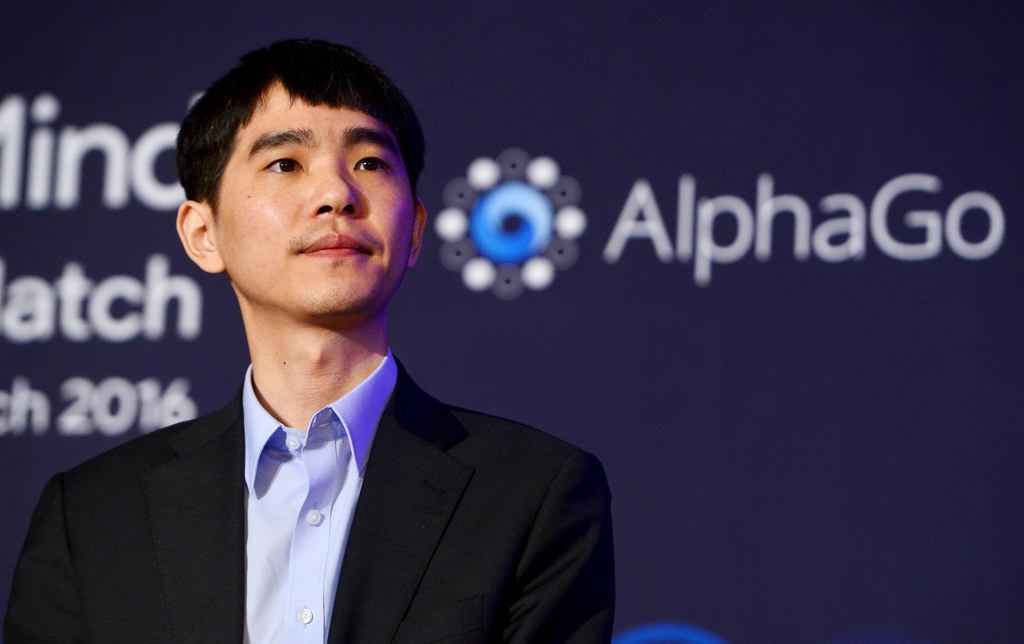
So its own designers probably don't know, really, how it works.
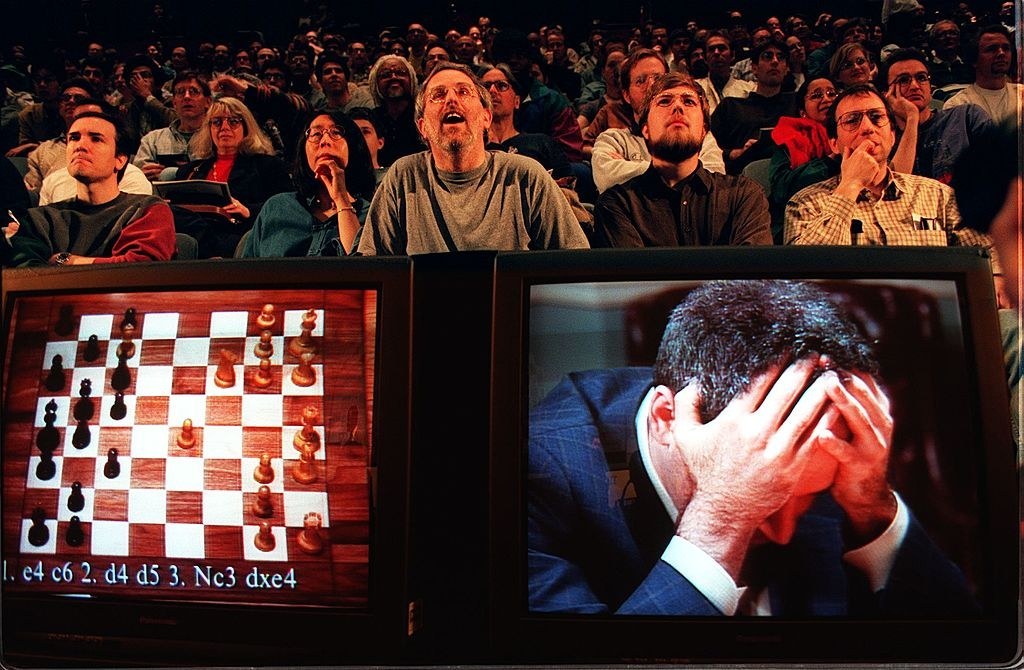
AlphaGo's victory has come as a major shock to the artificial intelligence community.
"People weren't expecting computer Go to be solved for 10 years," says Yudkowsky.
Even the AlphaGo team were shocked. Van den Driessche says: "We certainly were. We went very quickly from 'Let's see how well this works' to 'We seem to have a very strong player on our hands' to 'This player has become so strong that probably only a world champion can find its limits'."
While the way it learns is somewhat similar to how humans do, there are subtle but important differences.
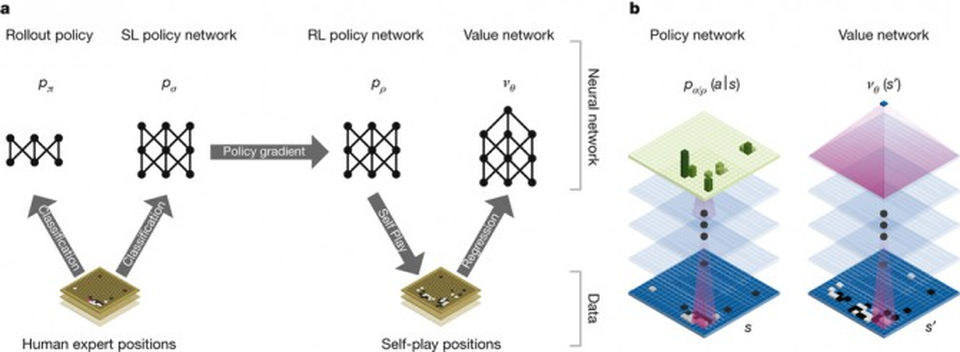
The way AlphaGo learns means that it has applications outside playing games.
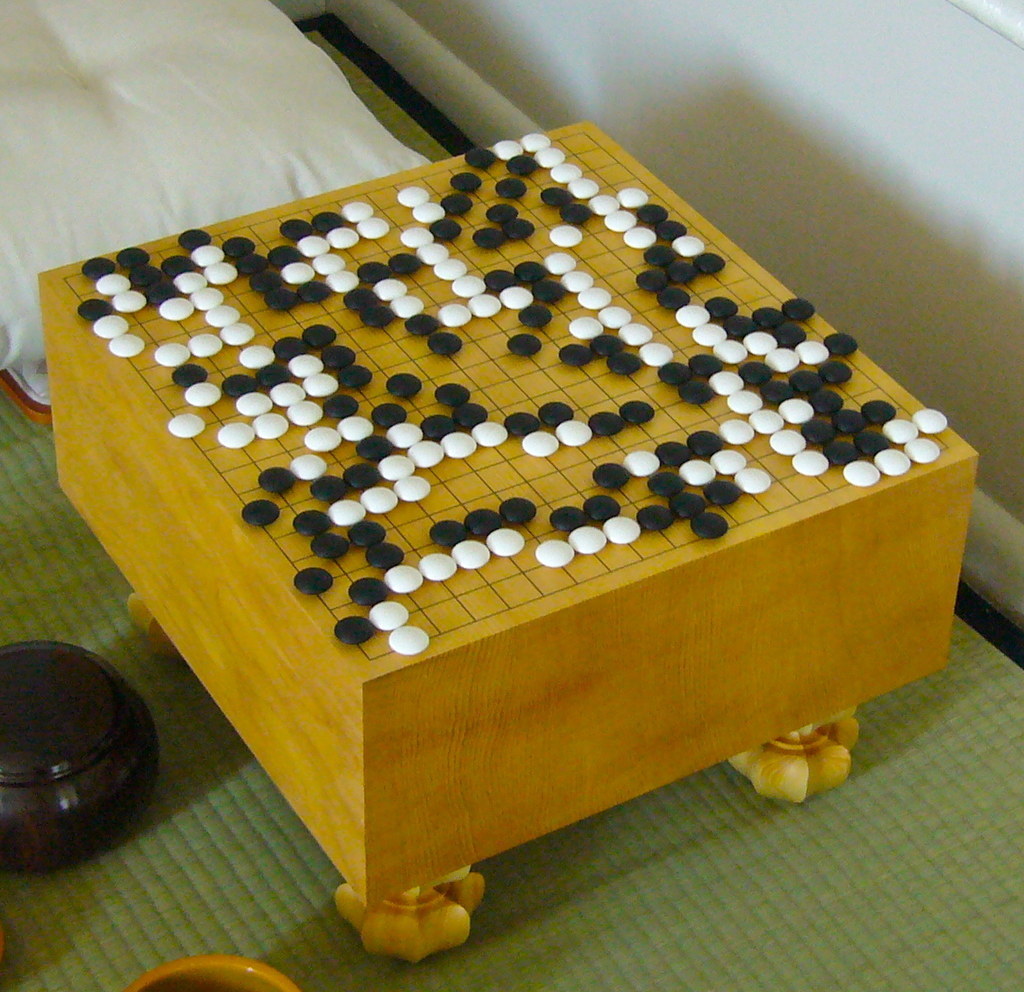
Artificial intelligence researchers say that this is a "sign of how far AI has come".
Computers like AlphaGo and Deep Blue, the machine that beat Garry Kasparov in 1997, are artificial intelligences, but they are intelligent in a highly specific way. The goal of some researchers is to develop an all-purpose intelligence, capable of solving all kinds of problems, as human brains are. That goal is known as "artificial general intelligence" (AGI).
AlphaGo's victory is a step along that road, says Shanahan, because of the generalisable way that it learns. He thinks that the techniques the AlphaGo team have used are the most promising route to AGI.
It's also a demonstration of just how powerful AI is now, and how quickly the field is moving, says Yudkowsky. "I'm not saying that AlphaGo in and of itself is going to lead to robots in 10 years," he says. "We just don't know about that. But AlphaGo is a sign of how far AI has come."
Although they warn there's a long way from here to true, human-level intelligence.
"Go is a tremendously complex game," says Shanahan. "But the everyday world is very, very much more complex." After all, he says, the real world contains Go, and chess, and driving cars. "The space of possible moves in the real world is truly huge. For example, the space of possible moves includes becoming a champion Go player."
He thinks that AGI is extremely unlikely in the next 10 years, but possible by 2050 and pretty likely by the end of the century. "This is not just a fantasy," he says. "We're talking about something that might actually affect our children, if not ourselves." Van den Driessche agrees, saying this is a "major milestone", but warning that human-level AGI is "still decades away".
"Nobody knows how long the road is [to AGI]," says Yudkowsky. "But we're pretty sure there's a long way left."
Still, they say, AlphaGo has shown that surprises happen. And AGI has the potential to be a big enough problem that it's worth paying attention now.
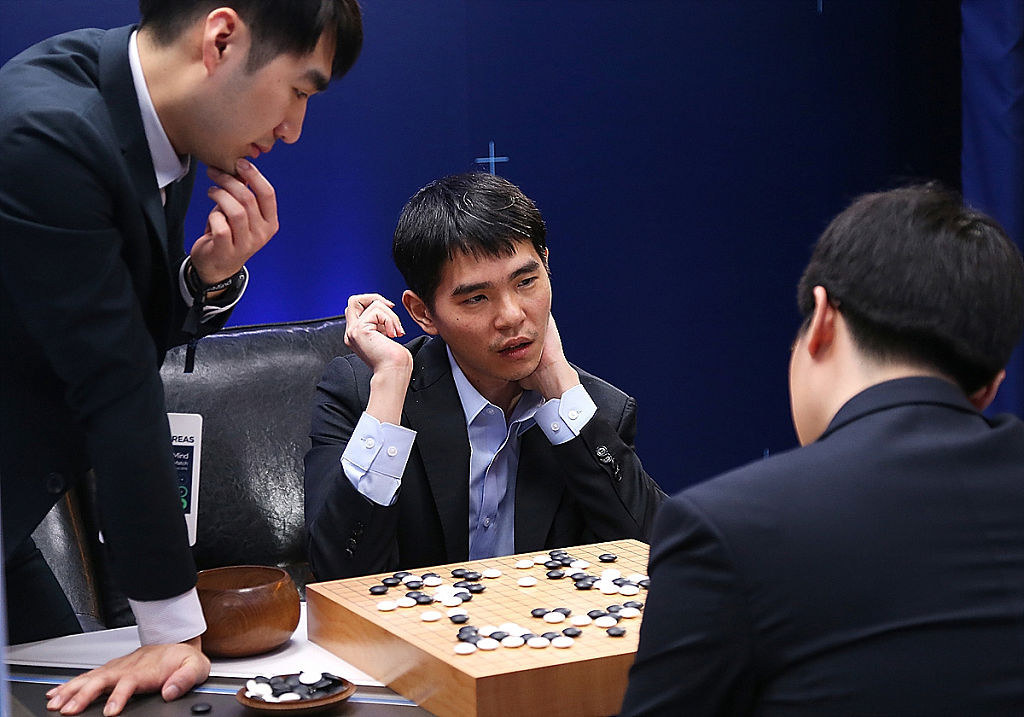
And AlphaGo has shown, too, that there's no reason to think that any future artificial intelligences need to be anything like us.
"In 1997, Garry Kasparov said that he sensed a kind of alien intelligence on the other side of the board," says Shanahan. "And I've noticed in the commentary on AlphaGo that some of the commentators thought that it had made some weak moves earlier on, but now they're not sure if it wasn't some clever plan for the end game. Sometimes an AI might solve things in a way that's quite different from how we might tackle things."
A future AGI, in a much more dramatic way, might not be "human" either. There's no reason to think it would have our desires, or even things that we'd call desires at all, says Shanahan. Bostrom has pointed out that there's no reason to think it would even be conscious.